While Generative AI offers great benefits like better decision-making, improved customer satisfaction, and lower operational risks, it also brings several challenges. It can create content, generate insights, and automate complex tasks, but implementing it can be complicated. Businesses often struggle with data quality, model reliability, and integration with existing systems, which can hinder its success. The enthusiasm around Generative AI can sometimes overshadow these important issues, creating a gap between its potential and real-world use.
In this article, we aim to tackle the challenges of using Generative AI development services in businesses. Explore common obstacles, including technical, ethical, and operational issues, and suggest ways to overcome them. By offering practical solutions, we provide insights to help organizations use Generative AI effectively. The goal is, therefore, to bridge the gap between theoretical benefits and practical use, so that it can be used by the firms to its full sustainable potential.
Benefits of Generative AI For Businesses
94% of leaders recognize the critical role of AI in the next five years. Generative AI holds immense potential for businesses. As per BCG, it increases productivity, personalized customer experiences, and accelerates R&D. Let’s delve into these benefits of implementing Generative AI further.
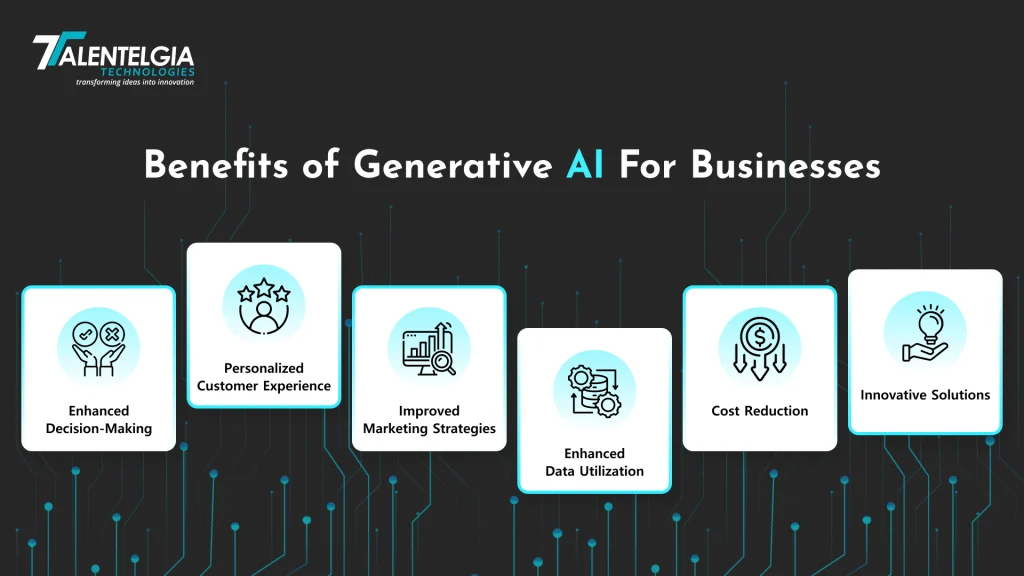
Key Challenges and Its Solutions: Generative AI in Business
Generative AI offers transformative potential across various industries, yet its implementation comes with significant challenges. We have mentioned key challenges and proposed targeted solutions. By addressing these challenges effectively, organizations can harness the full benefits of generative AI while minimizing risks and maximizing impact.
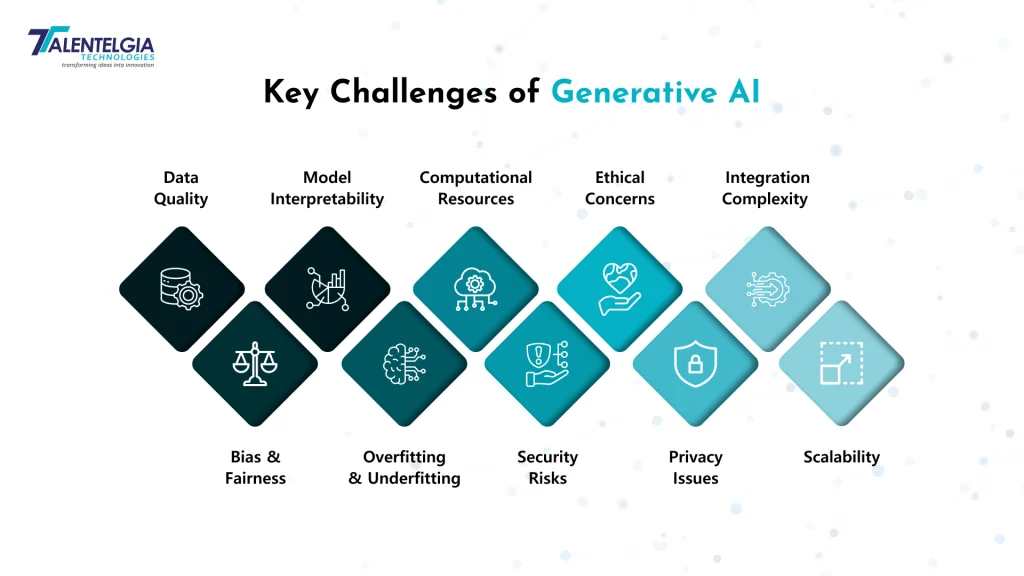
Data Quality
Challenge: Generative models generate inaccurate or undesirable outputs because of the use of poor quality or biased datasets. The issue arises from incomplete, dated, or unbalanced datasets that do not fully represent the problem domain and further result in a misguided model while learning and, therefore, in the predictions. Inaccuracies of this magnitude could be extremely distressing, mostly in sensitive applications such as healthcare apps or fintech apps where reliability is paramount. Inconsistencies in data collection alone, along with the effect of human error, further underline the importance of robust data preprocessing.
Solution: Data Augmentation – Enhance the quality and diversity of the training data through techniques such as data synthesis, augmentation, and filtering. By introducing varied and representative data, these methods can mitigate biases and improve model reliability, ensuring that the AI generates outputs that are more accurate and robust across different scenarios. Continual monitoring and updating of the data can further ensure ongoing quality and relevance. Additionally, employing rigorous data validation protocols and leveraging crowdsourcing for data labeling can enhance data quality.
Bias and Fairness
Challenge: Generative models can further replicate, if not increase, bias that exists in the training data, which can produce unfair or even discriminatory outcomes. The model may exaggerate contemporary stereotypes, omit minority groups, or perpetuate harmful practice in domains such as hiring or lending and law enforcement, among many others. This bias needs to be sorted out so that public trust is maintained and ethical applications of AI are ensured. Bias can also be subjected to legal liability and reputational damage; therefore, early detection and redress become imperative.
Bias Mitigation Techniques: Apply fairness-aware algorithms, reweighting training data, and diversity-enhancing strategies. These methods work by identifying biases in models and correcting them so that the output is fair; that is, the model has become balanced regarding judgment. Regular bias audit, further backed by engagement with stakeholders, continues to reshape fairness in AI. Inclusive design practices and a diverse developer team can also ensure a reduced bias.
Model Interpretability
Challenge: Generative models, especially deep neural networks, are hard to interpret and understand. This lack of transparency can hinder trust and make it hard for the user to diagnose errors, improve model performance, or ensure the model’s outputs adhere to ethical standards. In the absence of interpretability, compliance and user acceptance are difficult to achieve. In fact, this very black-box nature of models may lead to skepticism and resistance from stakeholders if they demand clarity regarding how decisions are made.
Solution: Explainable AI empowers stakeholders to understand the decision-making of the model with tools and techniques designed for transparency, such as feature importance analysis, visualization techniques, and interpretability frameworks. Such methods increase trust and give way to facilitate improvements in a more informed way. Incorporating user feedback into model development can also enhance interpretability. Developing user-friendly interfaces and documentation that explain model behavior in layman’s terms can further promote understanding and trust.
Overfitting/Underfitting
Challenge: The challenge with generative models is that they either fit too much in the training data, that is, become too specific and unable to generalize, or they underfit, being too general and missing major trends—a challenge in their creation of high-quality outputs. Addressing this trade-off is important to keeping performance on new, unseen data. Poor generalization may be the cause of the decrease in effectiveness and reliability of real-world applications. Overfitting can make a model very sensitive to small variations in the training dataset, while underfitting can cause oversimplification of the model, missing some of the critical insights.
Solution: Regularization Methods – Apply techniques like dropout, weight decay, and early stopping balance model complexity and generalization toward new, unseen data. These methods help prevent overfitting and underfitting, ensuring the model performs well across diverse situations. Periodic evaluation and tuning of hyper parameters can further optimize performance. Using cross-validation techniques and augmenting training datasets can also help in striking the right balance between model complexity and generalization.
Computational Resources
Challenge: Generative AI models often require substantial computational power and resources for training and inference. This high demand can lead to increased costs, longer development times, and limited accessibility for smaller organizations or researchers. Such requirements can also have a significant environmental impact due to high energy consumption. The need for specialized hardware and infrastructure can further constrain the scalability and deployment of generative models.
Solution: Efficient Algorithms – Utilize optimized algorithms, model pruning, and hardware acceleration (e.g., GPUs and TPUs) to manage and reduce computational demands. These approaches can make training and deploying generative models more efficient and cost-effective, broadening their accessibility and practicality. Exploring alternative, energy-efficient hardware options can further alleviate resource constraints. Leveraging cloud computing platforms and adopting distributed computing techniques can help in scaling computational resources dynamically.
Security Risks
Challenge: One of the challenges with generative AI lies in potential security risks because of adversarial attacks or misuse. A malicious entity may exploit vulnerabilities in the system, work around them, and manipulate outputs—a very harmful consequence for applications in areas such as security, finance, or content generation. The possibilities pose grave security and ethical challenges for AI-generated content to be used badly in making deep fakes or generating fake news.
Solution: Robust Security Measures – Security practices to be implemented include adversarial training, anomaly detection, access controls—which all help protect models against inimical activities—in model security. By having regular security audits and updating the security protocols, AI systems can also be better protected. Encourage responsible use and have clear guidelines, therefore minimizing the risks of misuse. The chances of withstanding an attack by an AI system will be increased through collaboration with cybersecurity experts and performing regular penetration testing.
Ethical Concerns
Challenge: This raises concerns with generative AI, for example, in creating deep fakes or propagating disinformation. Technologies can be used to deceive or manipulate people, and if there is such an aim, then the risks are huge to society. Guaranteeing AI applications are ethical and entails issues of transparency, accountability, and ways in which AI may further bad behavior or bias.
Solution: Ethical Guidelines –Set up and follow ethical guidelines and best practices of responsible AI, including transparency, accountability, and ethics review. Promotion of public awareness and engagement can help align AI development with societal values and norms. Developing regulatory frameworks can further enforce ethical practices. Engaging with ethicists, legal experts, and community stakeholders during the AI development process can ensure a more holistic approach to ethical considerations.
Privacy Issues
Challenge: Generative AI models can, unwillingly, leak sensitive information from the training dataset. Within that lies the potential for data leakage, and along with it, privacy breaches, losing user trust in any domain relevant to health or finance. This, therefore, underlines the need for maintaining user privacy to make sure that regulations concerning data protection and personal information safeguards are complied with.
Solution: Privacy-Preserving Techniques –Apply methods differential privacy and federated learning techniques to secure the privacy of every individual and be sure about the security of the data. Privacy audits can be conducted at regular intervals; one can also adhere to the regulations in the context of data protection for more security in case of sensitive information. Ensuring consent from the user and making data usage transparent can instill trust. In addition to that, privacy by design can be implemented through anonymization techniques to protect against privacy risks.
Integration Complexity
Challenge: Integrating generative AI models into existing systems and workflows can be complex and challenging. This difficulty can arise from compatibility issues, the need for significant modifications, and potential disruptions to current operations. The integration process may require retraining staff, updating legacy systems, and ensuring seamless interaction between new and existing components.
Solution: Modular Design – Develop models with modular architectures that allow for easier integration, customization, and scalability within existing infrastructures. Providing comprehensive documentation and support can facilitate smoother integration. Continuous testing and iterative improvement can ensure seamless adoption. Collaborating with IT teams and using standardized APIs can further simplify the integration process.
Scalability
Challenge: Scaling generative AI models to handle large datasets and growing user demands can be problematic. This challenge can lead to performance bottlenecks and reduced efficiency as the scale of operations increases. Ensuring that models can efficiently handle increased loads without degradation in performance is critical for their long-term viability.
Solution: Scalable Architectures – Design and deploy models using scalable architectures and cloud-based solutions that can efficiently handle increased data volumes and user requests. Leveraging distributed computing and advanced load balancing techniques can further enhance scalability. Regular performance monitoring and optimization can ensure sustained efficiency. Implementing microservices architecture and containerization can also help in scaling components independently, enhancing overall system flexibility and responsiveness.
Conclusion
Generative AI can yield transformative benefits in very diversified business domains. In optimized decision-making, improved customer satisfaction, and reduced operational risks—it also presents major challenges. Deployment of generative AI comes with complexities in data quality, model reliability, integration with existing systems, and scalability that pose critical problems for successful deployment and obscure the many potential benefits from AI technologies.
The key to meeting such challenges lies in a holistic approach: data augmentation for quality and variety, bias mitigation techniques for fairness, explainable AI for better model interpretability, regularization methods for balancing model performance. This would also include efficient algorithms for handling computational resources, robust security measures against threats, and ethical guidelines for the responsible use of AI. Privacy-preserving techniques secure sensitive information; modular design and scalable architectures guarantee easy integration and scaling.
If organizations turn their focus to the solution and give real-life examples of the benefits in reality, then they can be better at bridging the gap between theoretical benefits and practical implementation. This will help the businesses to effectively and sustainably unlock all the potential of Generative AI and drive innovation with a view to sustaining competitive advantage in the market. Mastering these pitfalls and strictly adhering to good ethical practices will enable the organization to maximize such impact that generative AI is capable of making while mitigating risks to have more informed and more beneficial outcomes.