The fast-paced evolution of the technological world has two innovations leading the transformation – namely, cloud computing and Edge AI. Cloud computing offers massive storage and computation, while Edge AI brings localized, real-time processing. Together, these two form a potent combination that revolutionizes industries to give way to advanced capabilities such as real-time decision-making, efficient resource utilization, and improved customer experiences.
Understanding these technologies’ synergy is important to leverage their combined power effectively. This article goes into their roles, importance, and applications, focusing on the critical role that cloud computing plays in enabling and enhancing Edge AI.
What is Cloud Computing?
Cloud computing refers to delivering computing services over the internet, often called “the cloud.” Users can access resources and applications on-demand without having direct active management of physical hardware.
Cloud computing delivers computing resources such as servers, storage, software, and databases over the internet. It helps organizations scale up their operations without investing in physical infrastructure. Thus, it is both cost-efficient and operationally agile.
Cloud platforms such as AWS, Microsoft Azure, and Google Cloud empower businesses by offering tools for large-scale data processing, analytics, and application deployment, which are essentials for modern AI-driven solutions.
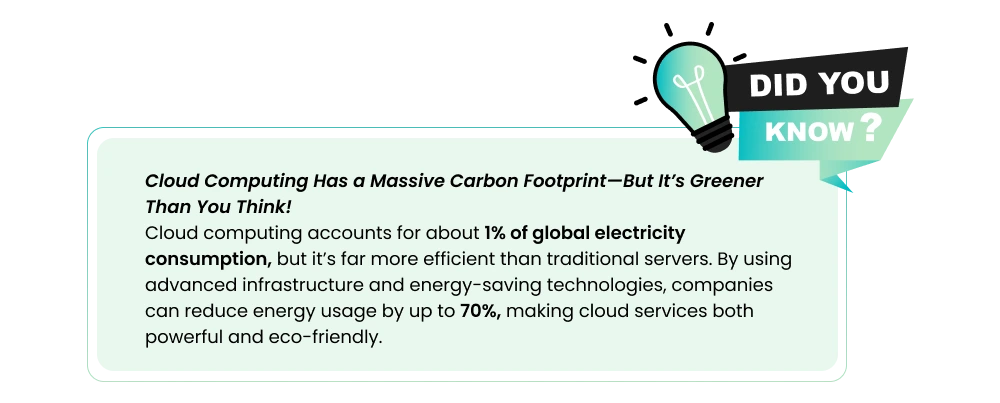
What is Edge AI?
Edge AI: It refers to the usage of artificial intelligence algorithms on edge devices or nodes. These can range from smartphones, IoT sensors, drones, and many other devices. Unlike traditional AI models, Edge AI does data processing locally, which eliminates latency, increases privacy, and thus allows the devices to remain functional even without the constant availability of the internet.
Example of Edge AI: Smart home assistants, autonomous vehicles, and wearable health monitor devices are some examples of using Edge AI.
This would further enhance both technologies’ strengths. Coupled together, cloud computing and Edge AI provide flexibility, scalability, and an efficient ecosystem for data collection, processing, and decision-making.
The key strengths of their integration include the following:
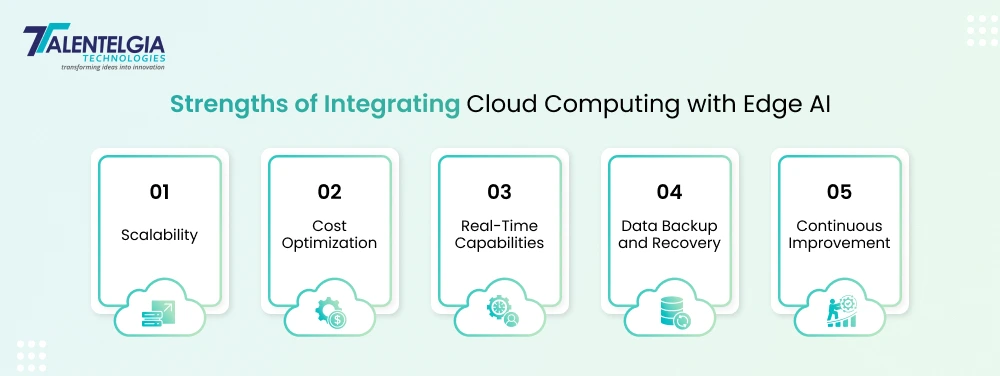
- Scalability: The cloud provides virtually limitless storage as well as computational power so that edge devices can easily scale their operations when they need to.
- Cost Optimization: Edge devices reduce the need for expensive local hardware by offloading intensive computations to the cloud.
- Real-Time Capabilities: Edge AI ensures instant responses, while the cloud provides deeper insights by analyzing aggregated data over time.
- Data Backup and Recovery: The cloud serves as a secure repository for edge-generated data, ensuring reliable backup and recovery.
- Continuous Improvement: AI models deployed on edge devices can be updated and improved with cloud-based training capabilities to ensure the accuracy and effectiveness of models.
Cloud Computing Role in Edge AI
Cloud computing is integral to Edge AI for many reasons. Here’s a closer view of the role of cloud computing in the same:
1. Model Training and Updates
These models require large amounts of datasets and computational power to be trained, which cloud platforms offer for this process. That frees up the edge devices for inference and real-time decision-making. It also facilitates frequent updates in the cloud for AI models to continue being effective and adaptable in scenarios they might not have previously experienced.
Example: In autonomous vehicles, cloud computing trains models in massive datasets gathered from sensors and cameras. These models are then transmitted to the edge devices in cars to operate in real-time.
2. Data Management and Storage
Edge devices generate enormous data daily. The cloud provides a scalable storage system, which guarantees the data is stored safely and accessible for later use. Cloud platforms also facilitate easy categorization and retrieval of data for compliance and auditing purposes.
For example, in healthcare applications, wearable devices collect patient health metrics such as heart rate and blood pressure. Such data is then transmitted to the cloud for long-term storage and analysis, allowing healthcare providers to monitor trends in patient health over time.
3. Augmenting Computational Power
The edge devices have limited processing power because of hardware limitations. For applications that need a lot of computing power, the cloud acts as an auxiliary processor. This ensures seamless operations even for resource-intensive applications.
Example: A drone in disaster management can perform preliminary analysis locally but offload high-resolution image processing to the cloud for deeper insights.
4. Real-Time Decision Support
While Edge AI shines with real-time processing, sometimes, some decisions require a more comprehensive analysis, and this should be better carried out in the cloud. Cloud computing supplements edge systems with added computational power, where timely and accurate decision-making is always achieved.
Example: Retail stores. Smart cameras make use of Edge AI for customer movement detection. On the other hand, the cloud systems analyze that data to forecast buying patterns and optimize the layout of the stores.
5. Security and Compliance
Data security is considered to be an utmost need for Edge AI applications. Cloud platforms also support such applications with heavy security aspects, such as encryption, access control, and monitoring threats. Compliance with data privacy regulations is also supported, including GDPR, HIPAA, and CCPA.
Example: Edge AI by financial institutions to detect fraud will also rely upon cloud computing for safeguarding sensitive data related to transactions and in compliance with regulations.
6. Orchestration and Monitoring
It is very challenging to manage a distributed network of edge devices. Cloud platforms provide central dashboards for monitoring the performance of devices, updating, and troubleshooting issues; this makes network management quite easy.
Example: In smart cities, IoT devices such as traffic lights and surveillance cameras are monitored and controlled using cloud-based tools, ensuring seamless operations.
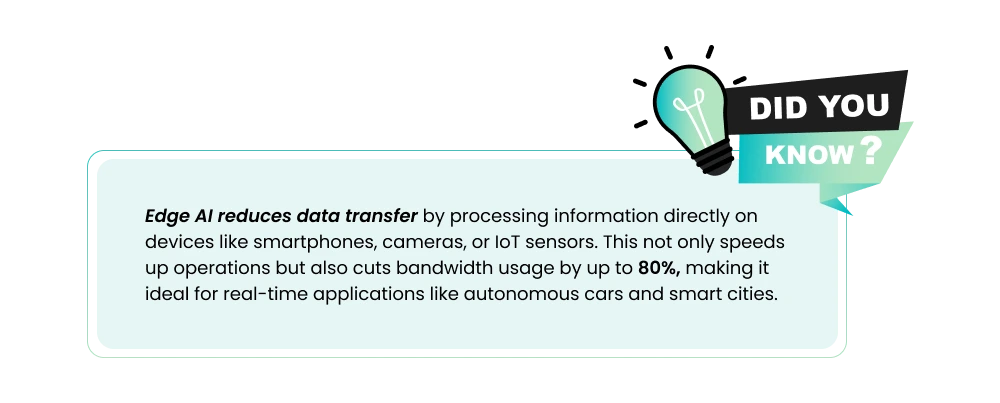
Key Technologies Enabling Cloud-Edge Collaboration
- IoT Devices: IoT devices are the basis for Edge AI since they collect and forward data. Examples include smart meters, wearable devices, and industrial sensors.
- 5G Connectivity: High-speed connectivity between edge devices and cloud, with ultra-low latency enabling real-time processing.
- Machine Learning Models: The cloud provides the computing resources for training and tuning machine learning models on edge devices.
Use Cases of Cloud-Edge AI Integration
1. Smart Cities
Smart city systems rely on Edge AI to monitor traffic, energy consumption, and waste management in real time. Cloud computing facilitates central coordination and long-term data analysis.
2. Healthcare Applications
Wearable devices use Edge AI for instant health monitoring. The data is stored and analyzed on the cloud platforms, offering deep insights and predictive analytics.
3. Autonomous Vehicles
Edge AI integration takes care of real-time navigation. Cloud computing manages route optimization and software updates, which keeps the vehicle safe and efficient.
4. Retail Industry
Smart shelves and cameras by Edge AI make the shopping experience personalized, while the cloud manages inventory and monitors sales data to understand sales trends.
5. Industrial Automation
Edge AI does quality checks and predictive maintenance in a factory, but the cloud just stores historical data and further refines the predictive models developed.
6. Environmental Monitoring
Edge devices, which are AI-enabled, analyze environmental data in real time, and the cloud consolidates this data to track climate patterns and develop mitigation strategies.
7. Agriculture
Edge AI systems in agriculture analyze soil quality and crop health, while cloud platforms provide long-term analysis and optimize farming practices based on weather patterns and market trends.
Conclusion
Thus, the collaboration between cloud computing and Edge AI unlocks new possibilities for smarter, faster, and more efficient systems. The synergy will only intensify because of the growth in IoT and 5G and will open up innovation throughout industries. This is more than a technological trend because it's the necessity through which organizations can thrive in an increasingly data-driven world.
Frequently Asked Questions(FAQs)
How does cloud computing address Edge AI challenges?
Cloud computing enhances Edge AI by providing scalable resources for model training, data storage, and complex processing tasks, overcoming the limitations of edge devices.
Can Edge AI operate without cloud computing?
The capability of Edge AI is enhanced much more by cloud computing since it can update models, store data, and apply advanced analytics.
What are the security concerns in Cloud-Edge AI, and how are they addressed?
It encompasses issues of security and data breaches, along with unauthorized access. The encryption, multi-factor authentication, and the advanced threat detection systems available in cloud platforms help overcome these problems.
How does cloud computing facilitate massive-scale Edge AI deployment?
Cloud computing offers centralized management tools, scalable resources, and real-time analytics, thus making it practicable to deploy and monitor huge networks of Edge AI.