Generative AI is transforming drug discovery. Large Language Models (LLMs) technology powering popular tools like ChatGPT and Google Gemini is now accelerating this process.
Generative AI models are now capable of devising novel molecule designs, predicting biological activity, and optimizing decisions. This is transforming the process of drug discovery to develop safer, more affordable drugs at record speed increases. The adoption of AI-driven tools is becoming more than just an advantage—it’s a necessity. Recent studies show that the drug discovery market is expected to reach USD 181.4 billion by 2032, growing from a value of USD 81.5 billion at a CAGR of 8.5% between 2023 and 2032, driven by these advancements. If this interests you, read below to find out more about it.
The Role of Generative AI in Drug Discovery
Businesses are actively seeking out AI development companies with robust machine learning capabilities to automate internal processes and drive innovation. In pharma, Generative AI—leveraging tools such as Large Language Models (LLMs) and neural networks—is revolutionizing the game.
It assists in early target identification, new molecule design, predicting clinical outcomes, and enhancing the overall drug development process. These AI tools accelerate research, lower costs, and make drug discovery more effective. Meanwhile, some startups are bringing in sophisticated techniques such as self-supervised learning and few-shot modeling, which can automate more phases with hardly any labeled data—reducing the time and expense of labor-intensive data annotation.
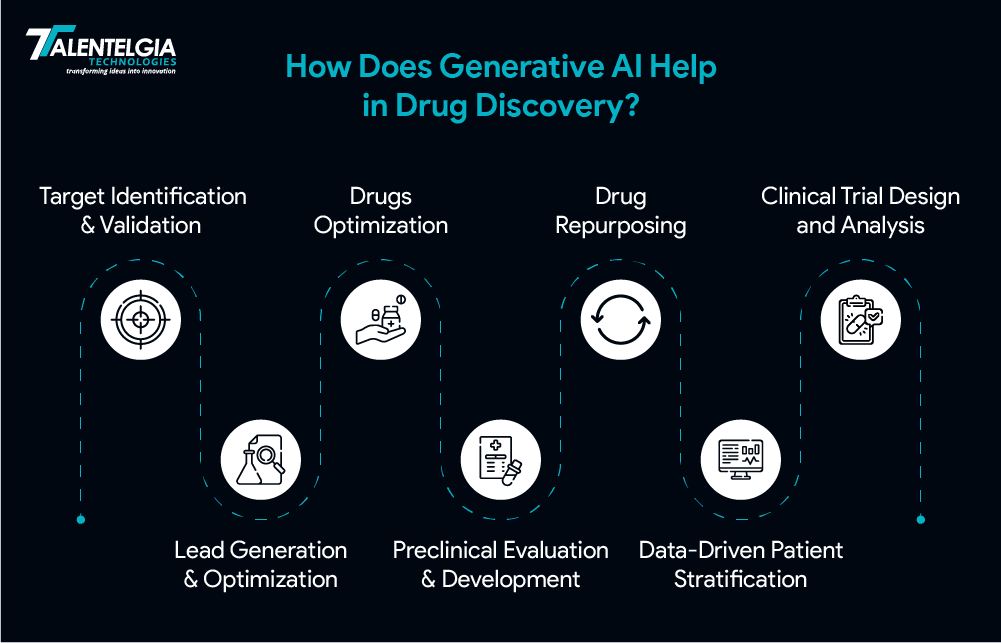
Target ID & Validation
The first step includes comprehending the disease and finding the possible biological targets, like proteins or genes, for drugs to interact with. This involves mining huge data collections of genes, protein structures, and disease mechanism information.
Generative AI helps by observing datasets for trends that researchers may not notice. It can identify which proteins are likely to be modified in a disease and propose the most promising targets to intervene. These targets are also validated by AI models, which simulate their role in disease progression and their response to perturbation.
Gather and Optimize Potential Leads
Finding molecules (also called the leads) that will bind effectively to the selected target is the next challenge. At this stage, scientists screen millions of compounds in the hope of finding a few that work.
With generative AI, this process reverses. Rather than screening compounds already in existence, it can create new ones from scratch. Generative models learn from known molecule-target interactions to generate new compounds that are predicted to bind strongly with the molecule of interest. The products generated can then undergo further optimization for efficacy, safety, and drug-like properties.
Molecule Simulations
These are important for understanding how a potential drug will behave in biological systems. The role of Generative AI in drug discovery is to improve that process by characterizing interactions at the atomic level. It can predict how a compound binds with a target protein, how stable it is in different pH or environments, and how it will be metabolized in the body.
This enables researchers to eliminate weak options early and focus on the molecules that exhibit strong binding affinity, low toxicity, and favorable properties. They replace or subsidise expensive lab experiments with simulations powered by AI. These accelerate early-stage evaluations and enhance decision-making.
De Novo Drug Design
De novo drug design is used to build new molecules from scratch rather than modify currently existing compounds.
Using specific biological targets and desired properties, generative AI models can be trained on huge chemical and biological datasets. This can create molecules atom by atom or fragment by fragment. Further, these AI-generated compounds are optimized for binding efficiency, solubility, toxicity profile, and other critical parameters.
This makes it easy to explore the chemical space well beyond what is currently known or explored. This allows researchers to identify truly novel drug candidates that would likely never be considered using traditional design approaches.
Virtual Screening
Virtual screening evaluates molecules using computational simulations instead of testing in lab. Generative AI enhances this approach by not just filtering but also forecasting which compounds are most likely to succeed according to past data.
It performs physical screenings at an impressive rate – it can rank molecules based on their estimated binding affinity, toxicity, and drug-likeness.
Other models take this further by simulating how molecules interact dynamically with targets under rapidly varying conditions. This increases the efficiency and accuracy of the process.
Compound Library Expansion
Compound libraries are collections of chemical structures and are a promising tool in drug discovery. Expanding these libraries was traditionally done through the manual synthesis and testing of new variants, which was a very time-consuming and costly process.
Generative AI automates this by creating new analogs around known compounds. It makes the required changes to core structures that might enhance their performance.
This approach adds to the chemical possibilities without needing a fresh start. AI can also deprioritize compounds that have biological activity but do not have compounds optimized for bioavailability, safety, ease of synthesis, etc. This increases the breadth and depth of libraries, improving the odds of finding a promising new drug.
Drug Optimization
Finding a lead is just the first stage of drug discovery. In many cases, the lead must go through multiple iterations before there is a stable and safe product. That is where optimization comes into play.
Generative AI models iteratively improve drug candidates by predicting how small changes impact the drug’s characteristics. Usually a slow and manual process, this iterative way of working can be accelerated as the role of AI in drug discovery becomes more advanced, increasing the likelihood of success in clinical trials.
Preclinical Assessment and Evaluation
Before any drug is tested on humans, it is extensively tested in the lab and on animals. Preclinical studies broadly evaluate its safety, efficacy, toxicity, and pharmacokinetics.
Generative AI assists in crafting superior experiments and dissecting intricate datasets from these studies. It can forecast side effects, mimic how a drug travels through the body, and identify biomarkers to monitor drug effectiveness. This lets researchers focus on the most promising options, saving researchers from expensive failures.
Drug Repurposing
Developing a new drug from scratch is expensive. But what if you could target a different disease using a drug that has already been approved?
This idea is the basis for drug repurposing. Generative AI accelerates repurposing by searching through vast amounts of biomedical databases and finding novel relationships between drugs, targets, and diseases. AI tools, for example, screened existing drugs for antiviral properties in the early months of the COVID-19 pandemic. This is especially important for rare or emergent diseases, where receiving information quickly can be critical.
Data-Driven Patient Stratification
Patients do not all respond to drugs the same way. Not all patients experience side effects, and some might not respond at all. These variations are at the core of personalized medicine. Generative AI helps identify patient subgroups for data-driven patient stratification by analyzing genomic, demographic, and clinical data. It can also predict which patients will benefit from a treatment and who will be more prone to experience side effects. This will help design better-targeted therapies and more effective clinical trials.
Clinical Trial Design and Analysis
Clinical trials are the longest and most expensive phase of drug development. They must be designed carefully to generate valid results. Generative AI can help improve trial design through simulating different trial designs, predicting outcomes, and identifying the right outcomes. During the trial, AI can analyze data continuously, notifying the researchers of any risks and generating insights that could lead to changes, enabling faster, safer, and more efficient trials.
Challenges of Gen AI in Drug Discovery
The success of AI in drug discovery relies on the inconsistency of the input data, the clarity of algorithms, and how well it fits into existing pharmaceutical pipelines.
Although generative AI in drug discovery is an emerging field, it also comes with potential challenges.
Check out the table below to learn more about these challenges and potential solutions.
Challenge | Solution |
---|---|
Data Quality and Availability | Improve data sharing and standardization across sources. |
Interpretability of AI Models | Use explainable AI tools to make model decisions transparent. |
Experimental Validation Bottlenecks | Prioritize high-confidence candidates and automate lab testing. |
Regulatory Uncertainty | Work with regulators to define clear AI evaluation standards. |
Integration into R&D Pipelines | Align AI tools with existing workflows and upskill teams. |
Also Read: Generative AI Challenges & Their Solutions
The Future of Generative AI in Drug Discovery
Generative AI in drug discovery is one of the most powerful models as its intricacies become more sophisticated and datasets richer.
Although generative AI isn’t going to replace scientists, it’s going to make them more efficient. It automates workflows and brings to the surface previously invisible patterns, allowing researchers to dedicate time to creative and strategic thinking. This makes the drug discovery process more intelligent, quicker, and agile.
- AI-designed drugs are moving more quickly and in higher volumes into clinical trials.
- Increased use of AI across the research and development continuum.
- Patient data will drive more targeted and personalized therapies.
- More collaboration between biologists, chemists, and Gen AI development services providers.
Conclusion
The function of Generative AI in drug development isn't simply the dance between algorithms, data, and compute—it's a matter of unlocking what's possible in medicine. Generative AI technology stack is assisting in developing life-saving medications that reach patients sooner than ever before. By examining vast data sets, designing new molecular configurations, and modeling outcomes with precision, Generative AI allows scientists to create treatments that are more personalized, more effective, and better targeted to individual patient conditions.
In addition to accelerating the process of development, Generative AI also lowers expenses, limits trial-and-error in the laboratory. Generative AI equips healthcare professionals with knowledge previously inaccessible. It enables everything from identifying new biomarkers to modeling drug effects—while delivering a precision and velocity that conventional methods cannot. And as the technology continues to mature, its impact on drug discovery and patient treatment will only intensify.
Talentelgia Technologies recognizes the pivotal role this innovation plays. With our extensive experience in AI/ML, Generative AI, and Large Language Models (LLMs), we assist pharmaceutical firms in creating smart, scalable solutions for their unique needs. Whether it's developing AI-driven platforms for molecule generation, incorporating self-supervised learning models for labeling data, or streamlining internal R&D workflows, our experts provide cutting-edge tools that speed up your drug discovery process—efficiently, securely, and with better outcomes.